基於稀疏控制點的多標記點雲標註
每天一分鐘,帶你讀遍機器人頂級會議文章
標題:Multi-Label Point Cloud Annotation by Selection of Sparse Control Points
作者:Riccardo Monica, Jacopo Aleotti, Michael Zillich, Markus Vincze
來源:3dv 2017 (International Conference on 3D Vision)
播音員:丸子
編譯:顏青松
歡迎個人轉發朋友圈;其他機構或自媒體如需轉載,後台留言申請授權
摘要
本文展示了一種容易使用的多標記點雲標註方法,即使用滑鼠交互選擇一系列稀疏控制點完成某目標的點雲標註(這也意味著多個控制點可以使用相同標註)。該方法以選中的控制點為基準,使用基於最短路徑樹的鄰域圖上的分割演算法。同時為方便用戶改正分割錯誤, 該方法還實時反饋分割效果。與之前的方法相比,本文中的方法支持對散亂點雲進行多標記標註。
本文中的方法已經被大量用戶使用,並且和常規的矩形框選標註方法進行了比較。實驗結果證明本文的方法更易使用,並且針對具有遮擋的複雜場景也能更快的進行分割和標註。
下圖展示了點雲標註軟體的界面。
下圖是在兩個數據集上,比較使用本文演算法和框選演算法的標註結果差異。第一行是使用的點雲數據,第二行是本文演算法的結果,第三行是框選式結果。
Abstract
This paper presents a user-friendly approach for multilabel point cloud annotation. The method requires the user to select sparse control points belonging to the objects through a mouse-based interface. Multiple control points may be assigned to the same label. The software utilizes the selected control points to perform a segmentation algorithm on the neighborhood graph, based on shortest path tree. The user is provided a real-time feedback about the result, and can correct segmentation errors. In contrast to previous work the method supports multi-label annotation of unorganized point clouds.
The method has been evaluated by multiple users and compared with a standard rectangle-based selection technique. Results indicate that the proposed method is perceived as easier to use, and that it allows a faster segmentation even in complex scenarios with occlusions
如果你對本文感興趣,想要下載完整文章進行閱讀,可以關注【泡泡機器人SLAM】公眾號(paopaorobot_slam)。

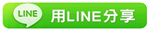
※基於語義分割的級聯光流法三維重建
※L2-Net:用深度學習方法設計塊描述子
TAG:泡泡機器人SLAM |