用於柵格地圖動態目標檢測的全卷積神經網路
每天一分鐘,帶你讀遍機器人頂級會議文章
標題:Fully Convolutional Neural Networks for Dynamic Object Detection in Grid Maps
作者:Florian Piewak, Timo Rehfeld, Michael Weber, and J. Marius Z?llner
來源:IEEE Intelligent Vehicles Symposium(IV2017)
播音員:四姑娘
編譯:陳建華
歡迎個人轉發朋友圈;其他機構或自媒體如需轉載,後台留言申請授權
摘要
大家好,今天為大家帶來的文章是——用於柵格地圖中動態目標檢測的全卷積神經網路,該文章發表於IV2017。
機器人領域廣泛使用柵格地圖來表示環境中的障礙物,並且將動態目標從靜態基礎設施中區分出來對於許多實際應用來說十分必要。在本文中,作者提出了一種使用深度卷積神經網路(CNN)的方法來推斷網格單元中是否存在移動目標。
圖1 本文方法的系統框架
對於上述問題,跟蹤方法通常是使用一個粒子濾波器來估計網格單元的速度,然後基於這個估計對每個網格單元做出是否存在移動目標的判斷。相比於跟蹤方法,作者的方法是將整個柵格地圖作為CNN的輸入圖像,對每個單元周圍的較大區域進行檢查,並且對柵格地圖的外觀結構進行了考慮後做出是否存在移動目標的判斷。
圖2 動態佔據網格地圖示例圖
在實驗部分,評價指標採用ROC曲線。相比於作者所參考的方法,本文的方法將性能從83.9%提高到97.2%。此外,作者還對運行時間進行了優化,並且優化版本能夠在輸出相同性能的情況下,僅需要10毫秒的執行時間。
圖3 動態佔據柵格地圖與動態目標檢測效果圖
圖4 不同輸入配置的ROC曲線
圖5 不同網路配置的ROC曲線
圖6 本文方法與參考方法的ROC曲線對比
Abstract
Grid maps are widely used in robotics to represent obstacles in the environment and differentiating dynamic objects from static infrastructure is essential for many practical applications. In this work, we present a method that uses a deep convolutional neural network (CNN) to infer whether grid cells are covering a moving object or not. Compared to tracking approaches, that use e.g. a particle filter to estimate grid cell velocities and then make a decision for individual grid cells based on this estimate, our approach uses the entire grid map as input image for a CNN that inspects a larger area around each cell and thus takes the structural appearance in the grid map into account to make a decision. Compared to our reference method, our concept yields a performance increase from 83.9% to 97.2%. A runtime optimized version of our approach yields similar improvements with an execution time of just 10 milliseconds.
如果你對本文感興趣,想要下載完整文章進行閱讀,可以關注【泡泡機器人SLAM】公眾號。

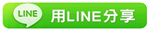
※能在任何錶面作畫的機器人;谷歌黑科技,深度學習視聽模式;喊你直接與書對話了!自動駕駛汽車的眼睛
※基於光場立體匹配的魯棒偽隨機場
TAG:泡泡機器人SLAM |