TensorFlow中的所有模型
概述
- 在本文中,我們將討論 TensorFlow 中當前可用的所有抽象模型,並描述該特定模型的用例以及簡單的示例代碼。 完整的工作示例源碼。
- 一個循環神經網路。
遞歸神經網路 簡稱 RNN
- 用例:語言建模,機器翻譯,詞嵌入,文本處理。
- 自從長短期記憶神經網路(LSTM)和門限循環單元(GRU)的出現,循環神經網路在自然語言處理中的發展迅速,遠遠超越了其他的模型。他們可以被用於傳入向量以表示字元,依據訓練集生成新的語句。這個模型的優點是它保持句子的上下文,並得出「貓坐在墊子上」的意思,意味著貓在墊子上。 TensorFlow 的出現讓創建這些網路變得越來越簡單。關於 TensorFlow 的更多隱藏特性可以從 Denny Britz 文章 中找到。
import tensorflow as tf
import numpy as np
# Create input data
X = np.random.randn(2, 10, 8)
# The second example is of length 6
X[1,6,:] = 0
X_lengths = [10, 6]
cell = tf.nn.rnn_cell.LSTMCell(num_units=64, state_is_tuple=True)
cell = tf.nn.rnn_cell.DropoutWrapper(cell=cell, output_keep_prob=0.5)
cell = tf.nn.rnn_cell.MultiRNNCell(cells=[cell] * 4, state_is_tuple=True)
outputs, last_states = tf.nn.dynamic_rnn(
cell=cell,
dtype=tf.float64,
sequence_length=X_lengths,
inputs=X)
result = tf.contrib.learn.run_n(
{"outputs": outputs, "last_states": last_states},
n=1,
feed_dict=None)
1
2
3
4
5
6
7
8
9
10
11
12
13
14
15
16
17
18
19
20
21
22
23
卷積網路
卷積網路
用例:圖像處理, 面部識別, 計算機視覺
卷積神經網路(Convolutional Neural Networks-簡稱 CNN )是獨一無二的,因為他可以直接輸入原始圖像,避免了對圖像複雜前期預處理。 CNN 用固定的窗口(下圖窗口為 3x3 )從左至右從上往下遍歷圖像。 其中我們稱該窗口為卷積核,每次卷積(與前面遍歷對應)都會計算其卷積特徵。
我們可以使用卷積特徵來做邊緣檢測,從而允許 CNN 描述圖像中的物體。
GIMP 手冊上邊緣檢測的例子
上圖使用的卷積特徵矩陣如下所示:
GIMP 手冊中的卷積特徵
下面是一個代碼示例,用於從 MNIST 數據集中識別手寫數字。
### Convolutional network
def max_pool_2x2(tensor_in):
return tf.nn.max_pool(
tensor_in, ksize=[1, 2, 2, 1], strides=[1, 2, 2, 1], padding="SAME")
def conv_model(X, y):
# reshape X to 4d tensor with 2nd and 3rd dimensions being image width and
# height final dimension being the number of color channels.
X = tf.reshape(X, [-1, 28, 28, 1])
# first conv layer will compute 32 features for each 5x5 patch
with tf.variable_scope("conv_layer1"):
h_conv1 = learn.ops.conv2d(X, n_filters=32, filter_shape=[5, 5],
bias=True, activation=tf.nn.relu)
h_pool1 = max_pool_2x2(h_conv1)
# second conv layer will compute 64 features for each 5x5 patch.
with tf.variable_scope("conv_layer2"):
h_conv2 = learn.ops.conv2d(h_pool1, n_filters=64, filter_shape=[5, 5],
bias=True, activation=tf.nn.relu)
h_pool2 = max_pool_2x2(h_conv2)
# reshape tensor into a batch of vectors
h_pool2_flat = tf.reshape(h_pool2, [-1, 7 * 7 * 64])
# densely connected layer with 1024 neurons.
h_fc1 = learn.ops.dnn(
h_pool2_flat, [1024], activation=tf.nn.relu, dropout=0.5)
return learn.models.logistic_regression(h_fc1, y)
1
2
3
4
5
6
7
8
9
10
11
12
13
14
15
16
17
18
19
20
21
22
23
24
前饋型神經網路
用例:分類和回歸
這些網路由一層層的感知器組成,這些感知器接收將信息傳遞到下一層的輸入,由網路中的最後一層輸出結果。 在給定層中的每個節點之間沒有連接。 沒有原始輸入和沒有最終輸出的圖層稱為隱藏圖層。
這個網路的目標類似於使用反向傳播的其他監督神經網路,使得輸入後得到期望的受訓輸出。 這些是用於分類和回歸問題的一些最簡單的有效神經網路。 下面代碼展示如何輕鬆地創建前饋型神經網路來分類手寫數字:
def init_weights(shape):
return tf.Variable(tf.random_normal(shape, stddev=0.01))
def model(X, w_h, w_o):
h = tf.nn.sigmoid(tf.matmul(X, w_h)) # this is a basic mlp, think 2 stacked logistic regressions
return tf.matmul(h, w_o) # note that we dont take the softmax at the end because our cost fn does that for us
mnist = input_data.read_data_sets("MNIST_data/", one_hot=True)
trX, trY, teX, teY = mnist.train.images, mnist.train.labels, mnist.test.images, mnist.test.labels
X = tf.placeholder("float", [None, 784])
Y = tf.placeholder("float", [None, 10])
w_h = init_weights([784, 625]) # create symbolic variables
w_o = init_weights([625, 10])
py_x = model(X, w_h, w_o)
cost = tf.reduce_mean(tf.nn.softmax_cross_entropy_with_logits(py_x, Y)) # compute costs
train_op = tf.train.GradientDescentOptimizer(0.05).minimize(cost) # construct an optimizer
predict_op = tf.argmax(py_x, 1)
# Launch the graph in a session
with tf.Session() as sess:
# you need to initialize all variables
tf.initialize_all_variables().run()
for i in range(100):
for start, end in zip(range(0, len(trX), 128), range(128, len(trX)+1, 128)):
sess.run(train_op, feed_dict={X: trX[start:end], Y: trY[start:end]})
print(i, np.mean(np.argmax(teY, axis=1) ==
sess.run(predict_op, feed_dict={X: teX, Y: teY})))
1
2
3
4
5
6
7
8
9
10
11
12
13
14
15
16
17
18
19
20
21
22
23
24
線性模型
用例:分類和回歸
線性模型根據 X 軸值的變化,併產生用於Y軸值的分類和回歸的最佳擬合線。 例如,如果你有一片區域房子的大小和價錢,那麼我們就可以利用線性模型來根據房子的大小來預測價錢。
需要注意的一點是,線性模型可以用於多個特徵。 例如在住房示例中,我們可以根據房子大小,房間數量和浴室數量以及價錢來構建一個線性模型,然後利用這個線性模型來根據房子的大小,房間以及浴室個數來預測價錢。
import numpy as np
import tensorflow as tf
import numpy as np
import tensorflow as tf
def weight_variable(shape):
initial = tf.truncated_normal(shape, stddev=1)
return tf.Variable(initial)
# dataset
xx = np.random.randint(0,1000,[1000,3])/1000.
yy = xx[:,0] * 2 + xx[:,1] * 1.4 + xx[:,2] * 3
# model
x = tf.placeholder(tf.float32, shape=[None, 3])
y_ = tf.placeholder(tf.float32, shape=[None])
W1 = weight_variable([3, 1])
y = tf.matmul(x, W1)
# training and cost function
cost_function = tf.reduce_mean(tf.square(tf.squeeze(y) - y_))
train_function = tf.train.AdamOptimizer(1e-2).minimize(cost_function)
# create a session
sess = tf.Session()
# train
sess.run(tf.initialize_all_variables())
for i in range(10000):
sess.run(train_function, feed_dict={x:xx, y_:yy})
if i % 1000 == 0:
print(sess.run(cost_function, feed_dict={x:xx, y_:yy}))
1
2
3
4
5
6
7
8
9
10
11
12
13
14
15
16
17
18
19
20
21
22
23
24
25
26
27
支持向量機
用例:目前只能用來做二進位分類
SVM 背後的一般思想是存在線性可分離模式的最佳超平面。 對於不可線性分離的數據,我們可以使用內核函數將原始數據轉換為新空間。 SVM 使分離超平面的邊界最大化。 它們在高維空間中非常好地工作,並且如果維度大於取樣的數量,SVM 仍然有效。
def input_fn():
return {
"example_id": tf.constant(["1", "2", "3"]),
"price": tf.constant([[0.6], [0.8], [0.3]]),
"sq_footage": tf.constant([[900.0], [700.0], [600.0]]),
"country": tf.SparseTensor(
values=["IT", "US", "GB"],
indices=[[0, 0], [1, 3], [2, 1]],
shape=[3, 5]),
"weights": tf.constant([[3.0], [1.0], [1.0]])
}, tf.constant([[1], [0], [1]])
price = tf.contrib.layers.real_valued_column("price")
sq_footage_bucket = tf.contrib.layers.bucketized_column(
tf.contrib.layers.real_valued_column("sq_footage"),
boundaries=[650.0, 800.0])
country = tf.contrib.layers.sparse_column_with_hash_bucket(
"country", hash_bucket_size=5)
sq_footage_country = tf.contrib.layers.crossed_column(
[sq_footage_bucket, country], hash_bucket_size=10)
svm_classifier = tf.contrib.learn.SVM(
feature_columns=[price, sq_footage_bucket, country, sq_footage_country],
example_id_column="example_id",
weight_column_name="weights",
l1_regularization=0.1,
l2_regularization=1.0)
svm_classifier.fit(input_fn=input_fn, steps=30)
accuracy = svm_classifier.evaluate(input_fn=input_fn, steps=1)["accuracy"]
1
2
3
4
5
6
7
8
9
10
11
12
13
14
15
16
17
18
19
20
21
22
23
24
25
26
27
深和寬的模型
用例:推薦系統,分類和回歸
深和寬模型在第二部分中有更詳細的描述,所以我們在這裡不會講解太多。 寬和深的網路將線性模型與前饋神經網路結合,使得我們的預測將具有記憶和泛化。 這種類型的模型可以用於分類和回歸問題。 這允許利用相對準確的預測來減少特徵工程。 因此,能夠結合兩個模型得出最好的結果。 下面的代碼片段摘自第二部分。
def input_fn(df, train=False):
"""Input builder function."""
# Creates a dictionary mapping from each continuous feature column name (k) to
# the values of that column stored in a constant Tensor.
continuous_cols = {k: tf.constant(df[k].values) for k in CONTINUOUS_COLUMNS}
# Creates a dictionary mapping from each categorical feature column name (k)
# to the values of that column stored in a tf.SparseTensor.
categorical_cols = {k: tf.SparseTensor(
indices=[[i, 0] for i in range(df[k].size)],
values=df[k].values,
shape=[df[k].size, 1])
for k in CATEGORICAL_COLUMNS}
# Merges the two dictionaries into one.
feature_cols = dict(continuous_cols)
feature_cols.update(categorical_cols)
# Converts the label column into a constant Tensor.
if train:
label = tf.constant(df[SURVIVED_COLUMN].values)
# Returns the feature columns and the label.
return feature_cols, label
else:
return feature_cols
m = build_estimator(model_dir)
m.fit(input_fn=lambda: input_fn(df_train, True), steps=200)
print m.predict(input_fn=lambda: input_fn(df_test))
results = m.evaluate(input_fn=lambda: input_fn(df_train, True), steps=1)
for key in sorted(results):
print("%s: %s" % (key, results[key]))
1
2
3
4
5
6
7
8
9
10
11
12
13
14
15
16
17
18
19
20
21
22
23
24
25
26
27
28
隨機森林
用例:分類和回歸
隨機森林模型中有很多不同分類樹,每個分類樹都可以投票來對物體進行分類,從而選出票數最多的類別。
隨機森林不會過擬合,所以你可以使用儘可能多的樹,而且執行的速度也是相對較快的。 下面的代碼片段是對鳶尾花數據集(Iris flower data set)使用隨機森林:
hparams = tf.contrib.tensor_forest.python.tensor_forest.ForestHParams(
num_trees=3, max_nodes=1000, num_classes=3, num_features=4)
classifier = tf.contrib.learn.TensorForestEstimator(hparams)
iris = tf.contrib.learn.datasets.load_iris()
data = iris.data.astype(np.float32)
target = iris.target.astype(np.float32)
monitors = [tf.contrib.learn.TensorForestLossMonitor(10, 10)]
classifier.fit(x=data, y=target, steps=100, monitors=monitors)
classifier.evaluate(x=data, y=target, steps=10)
1
2
3
4
5
6
7
8
9
貝葉斯強化學習(Bayesian Reinforcement Learning)
用例:分類和回歸
在 TensorFlow 的 contrib 文件夾中有一個名為 BayesFlow 的庫。 除了一個 REINFORCE 演算法的例子就沒有其他文檔了。 該演算法在 Ronald Williams 的論文中提出。
獲得的遞增 = 非負因子 * 強化偏移 * 合格的特徵
這個網路試圖解決立即強化學習任務,在每次試驗獲得強化值後調整權重。 在每次試驗結束時,每個權重通過學習率因子乘以增強值減去基線乘以合格的特徵而增加。 Williams 的論文還討論了使用反向傳播來訓練強化網路。
"""Build the Split-Apply-Merge Model.
Route each value of input [-1, -1, 1, 1] through one of the
functions, plus_1, minus_1. The decision for routing is made by
4 Bernoulli R.V.s whose parameters are determined by a neural network
applied to the input. REINFORCE is used to update the NN parameters.
Returns:
The 3-tuple (route_selection, routing_loss, final_loss), where:
- route_selection is an int 4-vector
- routing_loss is a float 4-vector
- final_loss is a float scalar.
"""
inputs = tf.constant([[-1.0], [-1.0], [1.0], [1.0]])
targets = tf.constant([[0.0], [0.0], [0.0], [0.0]])
paths = [plus_1, minus_1]
weights = tf.get_variable("w", [1, 2])
bias = tf.get_variable("b", [1, 1])
logits = tf.matmul(inputs, weights) + bias
# REINFORCE forward step
route_selection = st.StochasticTensor(
distributions.Categorical, logits=logits)
1
2
3
4
5
6
7
8
9
10
11
12
13
14
15
16
17
18
19
20
線性鏈條件隨機域 (Linear Chain Conditional Random Fields,簡稱 CRF)
用例:序列數據
CRF 是根據無向模型分解的條件概率分布。 他們預測單個樣本的標籤,保留來自相鄰樣本的上下文。 CRF 類似於隱馬爾可夫模型。 CRF 通常用於圖像分割和對象識別,以及淺分析,命名實體識別和基因發現。
# Train for a fixed number of iterations.
session.run(tf.initialize_all_variables())
for i in range(1000):
tf_unary_scores, tf_transition_params, _ = session.run(
[unary_scores, transition_params, train_op])
if i % 100 == 0:
correct_labels = 0
total_labels = 0
for tf_unary_scores_, y_, sequence_length_ in zip(tf_unary_scores, y, sequence_lengths):
# Remove padding from the scores and tag sequence.
tf_unary_scores_ = tf_unary_scores_[:sequence_length_]
y_ = y_[:sequence_length_]
# Compute the highest scoring sequence.
viterbi_sequence, _ = tf.contrib.crf.viterbi_decode(
tf_unary_scores_, tf_transition_params)
# Evaluate word-level accuracy.
correct_labels += np.sum(np.equal(viterbi_sequence, y_))
total_labels += sequence_length_
accuracy = 100.0 * correct_labels / float(total_labels)
print("Accuracy: %.2f%%" % accuracy)

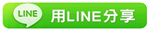
※DeepLearning-Ng編程中遇到的一些問題
※ubuntu16.04安裝TensorFlow的正確步驟
TAG:程序員小新人學習 |