NeurIPS 2018最佳論文發布:華為諾亞方舟實驗室等獲獎,加拿大實力凸顯
夏乙 問耕 發自 凹非寺
量子位 出品 | 公眾號 QbitAI
第31屆神經信息處理系統大會(NeurIPS 2018),今天正式開幕。
剛剛,4篇最佳論文獎、1篇時間檢驗獎悉數頒出。今年的最佳論文共有四篇。
其中有一篇的一作,來自華為諾亞方舟實驗室,另外這四篇論文以及作者的履歷,大多與加拿大的大學有關,凸顯了加拿大在人工智慧領域的實力。
這個大會,就是原來的人工智慧頂級會議NIPS,今年不僅有了全新簡稱NeurIPS,還有全新LOGO:左上角的N變成了一個Vr。
我們先來看看今年的獎項都發給了誰。
最佳論文s
下面我們分別介紹下這四篇論文。
第一篇被揭曉的是:Neural Ordinary Differential Equations
作者:Ricky T. Q. Chen, Yulia Rubanova, Jesse Bettencourt, David Duvenaud。
這篇論文來自加拿大多倫多大學Vector Institute,一作Ricky (Tian Qi) CHEN,本碩畢業於加拿大不列顛哥倫比亞大學,2017年迄今在多倫多大學讀博。
這篇論文開發了時間序列建模、監督學習和密度估計的新模型,研究了黑盒ODE求解器作為模型組件的使用。這些模型是自適應評估的,並允許明確控制計算速度和準確度之間的權衡。最後,作者推導了變數公式變化的瞬時版本,並開發了連續歸一化流程,而且可以拓展到更大的層尺寸。
摘要:
We introduce a new family of deep neural network models. Instead of specifying a discrete sequence of hidden layers, we parameterize the derivative of the hidden state using a neural network. The output of the network is computed using a black-box differential equation solver. These continuous-depth models have constant memory cost, adapt their evaluation strategy to each input, and can explicitly trade numerical precision for speed. We demonstrate these properties in continuous-depth residual networks and continuous-time latent variable models. We also construct continuous normalizing flows, a generative model that can train by maximum likelihood, without partitioning or ordering the data dimensions. For training, we show how to scalably backpropagate through any ODE solver, without access to its internal operations. This allows end-to-end training of ODEs within larger models.
論文地址:
https://arxiv.org/abs/1806.07366
第二篇最佳論文:Nearly Tight Sample Complexity Bounds for Learning Mixtures of Gaussians via Sample Compression Schemes
作者:Hassan Ashtiani、Shai Ben-David、Nicholas Harvey、Christopher Liaw、Abbas Mehrabian、Yaniv Plan。他們來自加拿大麥克馬斯特大學、滑鐵盧大學、不列顛哥倫比亞大學、麥吉爾大學等。
在這篇論文里,作者談到分散式學習和密度估計的一個核心問題,是表徵學習分布類的樣本複雜性。在二元分類中, Littlestone-Warmuth壓縮的組合概念被證明對學習是足夠和必要的,在這項工作中,作者發現新的相關分布壓縮足以進行分散式學習。
摘要:
We prove that Θ(kd2/ε2) samples are necessary and sufficient for learning a mixture of k Gaussians in Rd, up to error ε in total variation distance. This improves both the known upper bounds and lower bounds for this problem. For mixtures of axis-aligned Gaussians, we show that Θ(kd2/ε2) samples suffice, matching a known lower bound.
The upper bound is based on a novel technique for distribution learning based on a notion of sample compression. Any class of distributions that allows such a sample compression scheme can also be learned with few samples. Moreover, if a class of distributions has such a compression scheme, then so do the classes of products and mixtures of those distributions. The core of our main result is showing that the class of Gaussians in Rdhas an efficient sample compression.
論文地址:
https://papers.nips.cc/paper/7601-nearly-tight-sample-complexity-bounds-for-learning-mixtures-of-gaussians-via-sample-compression-schemes.pdf
第三篇最佳論文:Optimal Algorithms for Non-Smooth Distributed Optimization in Networks
作者:Kevin Scaman、Francis Bach、Sébastien Bubeck、Yin Tat Lee、Laurent Massoulié。他們來自華為諾亞方舟實驗室、INRIA、微軟研究院、華盛頓大學等機構。
在這篇論文中,作者在兩種情況下為非光滑和凸分散式優化提供了最優收斂速度:全局目標函數的Lipschitz連續性和局部個體函數的Lipschitz連續性。此外,作者還提供了第一個最優分散演算法:多步原始對偶(MSPD)。
摘要:
In this work, we consider the distributed optimization of non-smooth convex functions using a network of computing units. We investigate this problem under two regularity assumptions: (1) the Lipschitz continuity of the global objective function, and (2) the Lipschitz continuity of local individual functions. Under the local regularity assumption, we provide the first optimal first-order decentralized algorithm called multi-step primal-dual (MSPD) and its corresponding optimal convergence rate. A notable aspect of this result is that, for non-smooth functions, while the dominant term of the error is in O(1/√t), the structure of the communication network only impacts a second-order term in O(1/t), where t is time. In other words, the error due to limits in communication resources decreases at a fast rate even in the case of non-strongly-convex objective functions. Under the global regularity assumption, we provide a simple yet efficient algorithm called distributed randomized smoothing (DRS) based on a local smoothing of the objective function, and show that DRS is within a d1/4multiplicative factor of the optimal convergence rate, where d is the underlying dimension.
論文地址:
https://arxiv.org/abs/1806.00291
第四篇最佳論文:Non-Delusional Q-Learning and Value-Iteration
作者:Tyler Lu、Dale Schuurmans、Craig Boutilier,他們全都來自Google AI。其中一作Tyler Lu本碩畢業於加拿大滑鐵盧大學,博士畢業於多倫多大學。
在這篇論文中,作者指出妄想偏見(delusional bias)成為Q-Learning部署中的一個重要問題,而他們開發並分析了一個新的方法,可以完全消除妄想偏見。作者還提出了幾種針對大規模強化學習問題的實用啟發式方法,以減輕妄想偏差的影響。
摘要:
We identify a fundamental source of error in Q-learning and other forms of dynamic programming with function approximation. Delusional bias arises when the approximation architecture limits the class of expressible greedy policies. Since standard Q-updates make globally uncoordinated action choices with respect to the expressible policy class, inconsistent or even conflicting Q-value estimates can result, leading to pathological behaviour such as over/under-estimation, instability and even divergence. To solve this problem, we introduce a new notion of policy consistency and define a local backup process that ensures global consistency through the use of information sets—-sets that record constraints on policies consistent with backed-up Q-values. We prove that both the model-based and model-free algorithms using this backup remove delusional bias, yielding the first known algorithms that guarantee optimal results under general conditions. These algorithms furthermore only require polynomially many information sets (from a potentially exponential support). Finally, we suggest other practical heuristics for value-iteration and Q-learning that attempt to reduce delusional bias.
論文地址:
https://papers.nips.cc/paper/8200-non-delusional-q-learning-and-value-iteration
時間檢驗獎
今年的時間檢驗獎,頒發給2007年的一篇論文:The Tradeoffs of Large-Scale Learning。
作者:Leon Bottou、Olivier Bousquet,分別來自NEC美國實驗室和Google。
在這篇論文中,作者考慮到實例數量和計算時間的預算約束,發現了小規模學習系統和大規模學習系統的泛化性能之間存在質的差異。作者指出,大規模學習系統的泛化屬性,取決於估計過程的統計特性和優化演算法的計算特性。
摘要:
This contribution develops a theoretical framework that takes into account the effect of approximate optimization on learning algorithms. The analysis shows distinct tradeoffs for the case of small-scale and large-scale learning problems. Small-scale learning problems are subject to the usual approximation–estimation tradeoff. Large-scale learning problems are subject to a qualitatively different tradeoff involving the computational complexity of the underlying optimization algorithms in non-trivial ways.
論文地址:
https://papers.nips.cc/paper/3323-the-tradeoffs-of-large-scale-learning.pdf
想提高入選概率?放源代碼呀
除了頒獎之外,NeurIPS大會的組織者們還公布了不少有意思的數據。
首先就是肉眼已然可見的熱度。今年參加NeurIPS的,有8000多人,比去年稍多一點點。
之所以沒有暴漲,大概是受場地所限。畢竟,今年NeurIPS放票的時候,11分38秒就已經售罄,比火人節的票還難搶。不過大會的程序主席Hanna Wallach說,還是比碧昂絲演唱會賣得慢嘛。
不僅參加人數多,NeurIPS 2018收到的投稿也漲到了4854篇,不僅和去年相比大幅增長,還是今年ICML的兩倍。
從這些論文所在的領域來看,最火的出人意料,竟然並非大家都在談的深度學習,而是稍微寬泛一點的「演算法」。另外,強化學習的熱度雖然高的並不明顯,但比去年有大幅上升。
然後是論文的評審情況。
在4834名評審給出15000多條評審意見之後,NeurIPS 2018接收了1010篇論文,其中30篇oral、168篇spotlight、812篇poster。
雖然投稿多了不少,但是大會維持著和去年一樣的接收率,21%。
不過,這裡還有一點人生經驗需要傳授:如果你順便開源了代碼放出了數據,或者先把論文發到了arXiv上,中NeurIPS的概率就會有一定程度的提升。
不是量子位胡說的,有大會Co-Chair Hugo Larochelle列出的數據為證。他說,如果你提前把論文發在網上,還被至少一位評審者看到了,那麼接收率會達到34%;如果你提供了代碼或者數據,接收率會達到44%。
在今年的投稿中,有69%的作者說打算開源代碼,42%說要放出數據,56%提前把自己的論文發到了網上,其中又有35%被評審人看到了。
One More Thing
今年大會全部論文見:
Advances in Neural Information Processing Systems 31 (NIPS 2018) pre-proceedings
https://papers.nips.cc/book/advances-in-neural-information-processing-systems-31-2018
各大廠照例整理了自家的NeurIPS論文,下面是一系列傳送門:
https://ai.googleblog.com/2018/12/google-at-neurips-2018.html
https://research.fb.com/facebook-at-neurips-2018/
微軟
https://www.microsoft.com/en-us/research/event/neurips-2018/
騰訊
https://mp.weixin.qq.com/s/w6x9GCkcX-ZSWCR43ZTIfw
—完—
年度評選申請
加入社群
量子位AI社群開始招募啦,歡迎對AI感興趣的同學,在量子位公眾號(QbitAI)對話界面回復關鍵字「交流群」,獲取入群方式;
此外,量子位專業細分群(自動駕駛、CV、NLP、機器學習等)正在招募,面向正在從事相關領域的工程師及研究人員。
進專業群請在量子位公眾號(QbitAI)對話界面回復關鍵字「專業群」,獲取入群方式。(專業群審核較嚴,敬請諒解)
誠摯招聘
量子位正在招募編輯/記者,工作地點在北京中關村。期待有才氣、有熱情的同學加入我們!相關細節,請在量子位公眾號(QbitAI)對話界面,回復「招聘」兩個字。

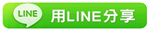
※自動駕駛高峰激辯:寒冬還有多遠,晶元路線之爭,人才缺乏待解
TAG:量子位 |