實時單人姿態估計,在自己手機上就能實現 : ) 安卓和iOS都可以哦~
選自GitHub
作
者:
edvardHua
參與:路
本文介紹了如何使用 TensorFlow 在智能機上(包括安卓和 iOS 設備)執行實時單人姿態估計。
GitHub 地址:https://github.com/edvardHua/PoseEstimationForMobile
該 repo 使用 TensorFlow 實現 CPM 和 Hourglass 模型。這裡未使用常規的卷積,而是在模型內部使用了反向卷積(又叫 Mobilenet V2),以便執行實時推斷。
註:你可以修改網路架構,來訓練更高 PCKh 的模型。架構地址:https://github.com/edvardHua/PoseEstimationForMobile/tree/master/training/src
該庫包含:
訓練 CPM 和 Hourglass 模型的代碼;
安卓 demo 的源代碼;
IOS demo 的源代碼。
下面的 gif 是在 Mi Mix2s 上截取的(~60 FPS)
你可以下載以下 apk,在自己的設備上進行測試。
PoseEstimation-Mace.apk:https://raw.githubusercontent.com/edvardHua/PoseEstimationForMobile/master/release/PoseEstimation-Mace.apk
PoseEstimation-TFlite.apk:https://raw.githubusercontent.com/edvardHua/PoseEstimationForMobile/master/release/PoseEstimation-TFlite.apk
訓練
依賴項
Python3
TensorFlow >= 1.4
Mace
數據集
訓練數據集:https://drive.google.com/open?id=1zahjQWhuKIYWRRI2ZlHzn65Ug_jIiC4l
將其解壓縮,獲取以下文件結構:
# root @ ubuntu in ~/hdd/ai_challenger 1
$ tree -L
├── ai_challenger_train.json
├── ai_challenger_valid.json
├── train
└── valid
該訓練數據集僅包含單人圖像,數據來源是 AI Challenger 競賽。共包含 22446 個訓練樣本和 1500 個測試樣本。
該 repo 作者使用 tf-pose-estimation 庫中的數據增強代碼將標註遷移為 COCO 格式。tf-pose-estimation 庫:https://github.com/ildoonet/tf-pose-estimation
超參數
訓練步驟中,使用 experiments 文件夾中的 cfg 文件傳輸超參數。
以下是 mv2_cpm.cfg 文件的內容:
"mv2_cpm" False "/root/hdd/ai_challenger" "/root/hdd/" "0, 1, 2" 8 1000[Train]
model:
checkpoint:
datapath:
imgpath:
visible_devices:
multiprocessing_num:
max_epoch:
lr:
"0.001"
batchsize:
5
decay_rate:
0.95
input_width:
192
input_height:
192
n_kpoints:
14
scale:
2
modelpath:
"/root/hdd/trained/mv2_cpm/models"
logpath:
"/root/hdd/trained/mv2_cpm/log"
num_train_samples:
20000
per_update_tensorboard_step:
500
per_saved_model_step:
2000
pred_image_on_tensorboard:
True
該 cfg 文件覆蓋模型的所有參數,在 network_mv2_cpm.py 中仍有一些參數。
使用 nvidia-docker 訓練
通過以下命令構建 docker:
cd training/docker
docker build -t single-pose .
或者
docker pull edvardhua/single-pose
然後運行以下命令,訓練模型:
6006 6006nvidia-docker run -it -d
-v <dataset_path>:/data5 -v <training_code_path>/training:/workspace
-p
-e PARAMETERS_FILE=experiments/mv2_cpm.cfg edvardhua/single-pose
此外,它還在 port 6006 上創建了 tensorboard。確保安裝了 nvidia-docker。
按一般方法訓練
1. 安裝依賴項:
cd training
pip3 install -r requirements.txt
還需要安裝 cocoapi (https://github.com/cocodataset/cocoapi)。
2. 編輯 experiments 文件夾中的參數文件,它包含幾乎所有超參數和訓練中需要定義的其他配置。之後,傳輸參數文件,開始訓練:
cd training
python3 src/train.py experiments/mv2_cpm.cfg
在 3 張英偉達 1080Ti 顯卡上經過 12 個小時的訓練後,該模型幾乎收斂。以下是對應的 tensorboard 圖。
基準(PCKh)
運行以下命令,評估 PCKh 值。
-360000.python3 src/benchmark.py --frozen_pb_path=hourglass/model
--anno_json_path=/root/hdd/ai_challenger/ai_challenger_valid.json
--img_path=/root/hdd
--output_node_name=hourglass_out_3
預訓練模型
CPM:https://github.com/edvardHua/PoseEstimationForMobile/tree/master/release/cpm_model
Hourglass:https://github.com/edvardHua/PoseEstimationForMobile/tree/master/release/hourglass_model
安卓 demo
由於 mace 框架,你可以使用 GPU 在安卓智能機上運行該模型。
按照以下命令將模型轉換為 mace 格式:
# You transer hourglass or cpm model by changing `yml` file.cd <your-mace-path>
python tools/converter.py convert --config=<PoseEstimationForMobilePath>/release/mace_ymls/cpm.yml
然後根據 mace 文檔的說明,將模型集成到安卓設備中。
至於如何調用模型、解析輸出,可以參見安卓源代碼:https://github.com/edvardHua/PoseEstimationForMobile/tree/master/android_demo。
一些晶元的平均推斷時間基準如下所示:
以下是該 repo 作者構建該 demo 的環境:
操作系統:macOS 10.13.6(mace 目前不支持 windows)
Android Studio:3.0.1
NDK 版本:r16
在構建 mace-demo 時,不同環境可能會遇到不同的錯誤。為避免這種情況,作者建議使用 docker。
docker pull registry.cn-hangzhou.aliyuncs.com/xiaomimace/mace-dev-lite
docker run -it
--privileged -d --name mace-dev
--net=host
-v to/you/path/PoseEstimationForMobile/android_demo/demo_mace:/demo_mace
registry.cn-hangzhou.aliyuncs.com/xiaomimace/mace-dev-lite
docker run -it --privileged -d --name mace-dev --net=host
-v to/you/path/PoseEstimationForMobile/android_demo/demo_mace:/demo_mace
registry.cn-hangzhou.aliyuncs.com/xiaomimace/mace-dev-lite
# Enter to docker
docker
exec
-it mace-dev bash# Exec command inside the dockercd /demo_mace && ./gradlew build
或者將模型轉換為 tflite:
# Convert to frozen pb.cd training 192 # If you update tensorflow to 1.9, run following command. "image" "Convolutional_Pose_Machine/stage_5_out" "./" # Convert to tflite.# See https://github.com/tensorflow/tensorflow/blob/master/tensorflow/docs_src/mobile/tflite/devguide.md for more information. "1,192,192,3" "image" "Convolutional_Pose_Machine/stage_5_out"
python3 src/gen_frozen_pb.py
--checkpoint=<you_training_model_path>/model-xxx --output_graph=<you_output_model_path>/model-xxx.pb
--size=
python3 src/gen_tflite_coreml.py
--frozen_pb=forzen_graph.pb
--input_node_name=
--output_node_name=
--output_path=
--type=tflite
bazel-bin/tensorflow/contrib/lite/toco/toco
--input_file=<you_output_model_path>/model-xxx.pb
--output_file=<you_output_tflite_model_path>/mv2-cpm.tflite
--input_format=TENSORFLOW_GRAPHDEF --output_format=TFLITE
--inference_type=FLOAT
--input_shape=
--input_array=
--output_array=
然後,將 tflite 文件放在 android_demo/app/src/main/assets 中,修改 ImageClassifierFloatInception.kt 中的參數。
in in is 192 192 96 96 "mv2-cpm.tflite" 4............// parameters need to modify
fun create(
activity: Activity,
imageSizeX: Int =
imageSizeY: Int =
outputW: Int =
outputH: Int =
modelPath: String =
numBytesPerChannel: Int =
): ImageClassifierFloatInception =
ImageClassifierFloatInception(
activity,
imageSizeX,
imageSizeY,
outputW,
outputH,
modelPath,
numBytesPerChannel)............
最後,將該項目導入 Android Studio,在智能機設備上運行。
iOS Demo
首先,將模型轉換為 CoreML 模型:
# Convert to frozen pb.cd training 192 # Run the following command to get mlmodel "image" "Convolutional_Pose_Machine/stage_5_out" "./"
python3 src/gen_frozen_pb.py
--checkpoint=<you_training_model_path>/model-xxx --output_graph=<you_output_model_path>/model-xxx.pb
--size=
python3 src/gen_tflite_coreml.py
--frozen_pb=forzen_graph.pb
--input_node_name=
--output_node_name=
--output_path=
--type=coreml
然後,按照 PoseEstimation-CoreML 中的說明來操作(https://github.com/tucan9389/PoseEstimation-CoreML)。
本文為機器之心編譯,
轉載請聯繫本公眾號獲得授權
。?------------------------------------------------
加入機器之心(全職記者 / 實習生):hr@jiqizhixin.com
投稿或尋求報道:
content
@jiqizhixin.com廣告 & 商務合作:bd@jiqizhixin.com

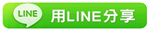
※成真?英國警方準備使用AI預測犯罪
※2018人工智慧期末考試複習資料(一):學術篇
TAG:機器之心 |